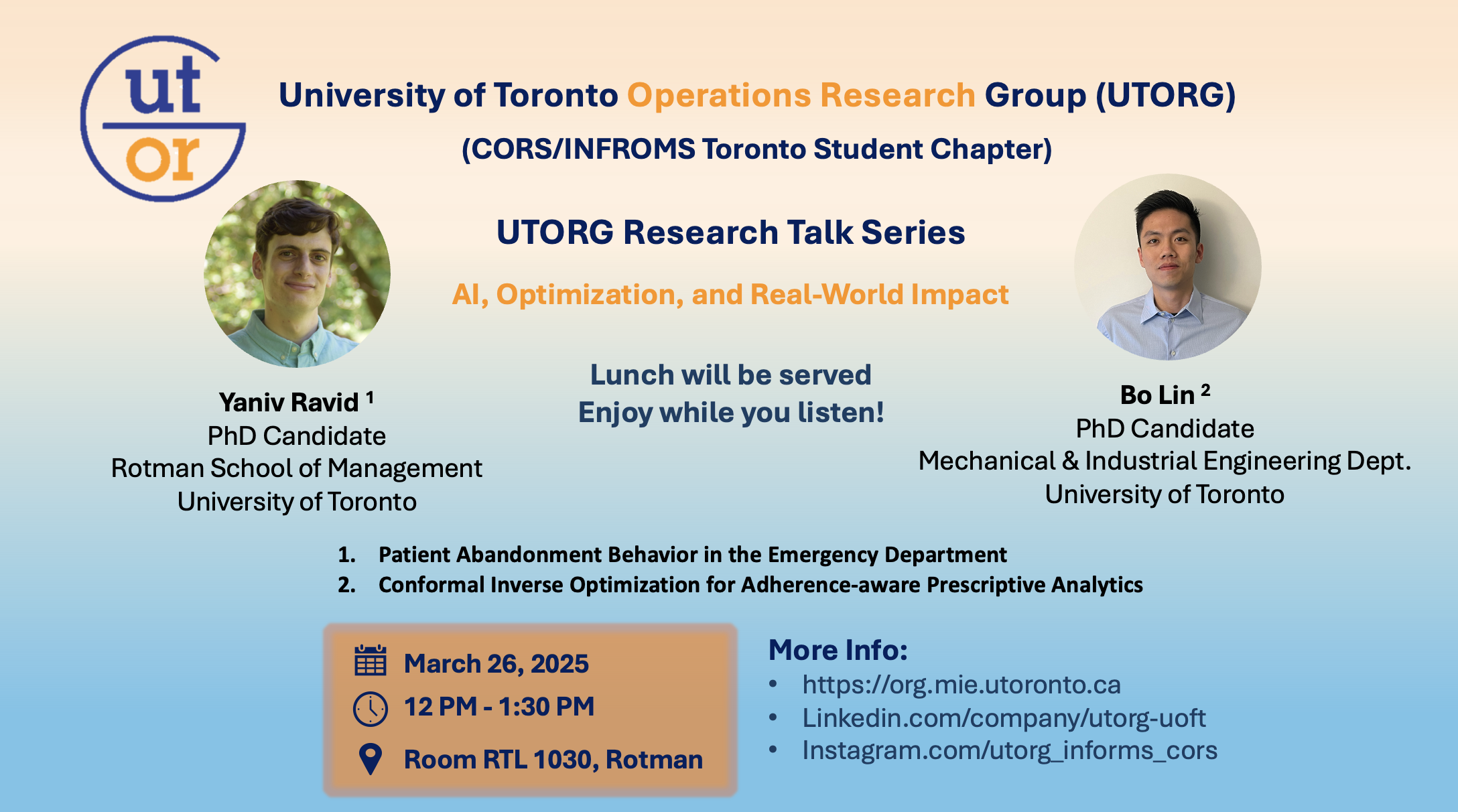
Join us for insightful presentations by two of our fellow students, and enjoy a delicious lunch served during the talks.
Event Details
Date: Wednesday, March 26
Time: 12:00 PM – 1:30 PM
Location: RTL (Rotman Lower Level) 1030, Rotman School of Management, University of Toronto
Please Register Here so we can prepare the right amount of food and seating. Don’t worry if you forget to register—you’re still welcome to join us!
First Talk : Patient Abandonment Behavior in the Emergency DepartmentÂ
Abstract
Study objective: In this study, we developed and validated machine learning models to predict emergency department (ED) patients’ tendency to leave without being seen (LWBS). We used patient as well as system characteristics in our models which dynamically updated these risk levels as patients spent more time waiting. We applied these models to an experimental cohort, and show that it misses fewer LWBS cases than previous models that do not dynamically update their predictions. Methods: A dataset of 150,959 patient arrivals at an ED of a large academic institution was collected over a period of 24 months from 2017 to 2019. We used the first 18 months of data to train and validate the machine learning models, with the final six months serving as an experimental period. The models were applied to the experimental period and their ability to identify real LWBS cases was measured. Results: The models achieved an area under the receiver operating characteristic curve (AUC) of 0.86 when dynamically updating LWBS risk levels over time. This was in contrast to an AUC of 0.80 for the static model from past literature that does not update the score after the moment of arrival. Among the experimental cohort, the dynamic model also showed the ability to reduce the number of missed LWBS cases by approximately 50% as compared to the static model. Conclusion: Prediction models were created to accurately identify patients who will LWBS, as well as when during their wait they are most likely to LWBS. When tested on a cohort that was not used in the training stages, the models illustrated the importance of updating patients’ risk of LWBS as their wait continued.
Bio
Yaniv Ravid is a 4th year PhD student at the Operations Management and Statistics department at Rotman School of Management. His research utilizes data analytics to better address operational risks and business systems disruptions. Before his doctoral studies, Yaniv worked in the tech industry as a software consultant and data security specialist. He obtained his Master of Engineering in Operations Research and Information Engineering from Cornell University.
Second Talk : Conformal Inverse Optimization for Adherence-aware Prescriptive AnalyticsÂ
Abstract
Inverse optimization is increasingly used to estimate unknown parameters in an optimization model based on decision data. We show that such a point estimate alone is insufficient in a prescriptive setting where the estimated parameters are used to prescribe new decisions. The resulting decisions may be low-quality and misaligned with human intuition and thus are unlikely to be adopted. To tackle this challenge, we propose a novel decision recommendation pipeline, which seeks to learn an uncertainty set for the unknown parameters and then solve a robust optimization model to prescribe new decisions. We show that the suggested decisions can achieve bounded optimality gaps, as evaluated using both the ground-truth parameters and human perceptions. Our method demonstrates strong empirical performance compared to the standard inverse optimization pipeline. Finally, we perform a case study where we apply this new pipeline to provide delivery route recommendations in Toronto, Canada. Our approach achieves a significantly higher delivery path adherence rate than current industry practices without compromising service quality. Moreover, our method provides a better trade-off between absolute and perceived decision quality than baselines under various realistic scenarios, including cases with model mis-specification and data scarcity.
Bio
Bo Lin is a PhD candidate at the University of Toronto. His research centres around AI for sustainable cities, with a focus on urban mobility to date. His research has been widely referenced in policy documents and mainstream media, including CBC News, Toronto Star, U of T News, and Uber Engineering Blog. His work has been recognized with several awards, including the INFORMS TSL Best Student Paper Award, runner-up for the CORS Best Student Paper Award, and finalist nominations for the INFORMS Service Science Best Cluster Paper Award and INFORMS Data Mining Best Theoretical Paper Award. In 2023, he interned as an Applied Scientist on Uber’s Core Analytics and Science team.
We look forward to seeing you!